The NVIDIA Titan V Preview - Titanomachy: War of the Titans
by Ryan Smith & Nate Oh on December 20, 2017 11:30 AM EST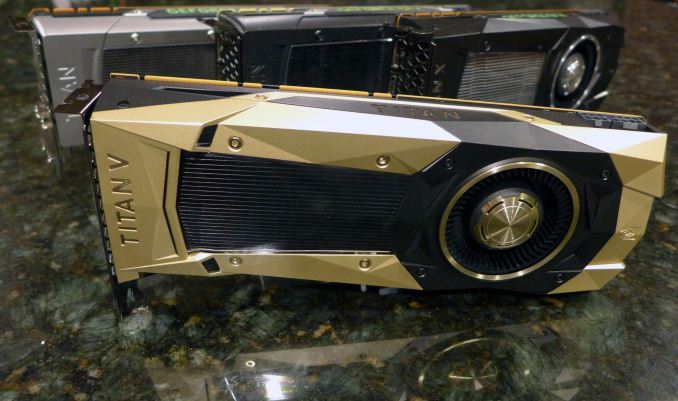
Over the years we at AnandTech have had the interesting experience of covering NVIDIA’s hard-earned but none the less not quite expected meteoric rise under the banner of GPU computing. Nearly a decade ago CEO Jen-Hsun Huang put the company on a course to invest heavily in GPUs as compute accelerators, and while it seemed likely to pay off – the computing industry has a long history of accelerators – when, where, and how ended up being a lot different than Huang was first expecting. Instead of the traditional high performance computing market, the flashpoint for NVIDIA’s rapid growth has been in neural networking, a field that wasn’t even on the radar 10 years ago.
I bring this up because in terms of NVIDIA’s product line, I don’t think there’s a card that better reflects NVIDIA’s achievements and shifts in compute strategy than the Titan family. Though originally rooted as a sort of flagship card of the GeForce family that lived a dual life between graphics and compute, the original GTX Titan and its descendants have instead transitioned over the years into an increasingly compute-centric product. Long having lost its GeForce branding but not the graphical capabilities, the Titan has instead drifted towards becoming a high performance workstation-class compute card. Each generation of the Titan has pushed farther and farther towards compute, and if we’re charting the evolution of the Titan, then NVIDIA’s latest Titan, the NVIDIA Titan V, may very well be its biggest jump yet.
Launched rather unexpectedly just two weeks ago at the 2017 Neural Information Processing Systems conference, the NVIDIA Titan V may be the most important Titan yet for the company. Not just because it’s the newest, or because it’s the fastest – and oh man, is it fast – or even because of the eye-popping $3000 price tag, but because it’s the first card in a new era for the Titan family. What sets the Titan V apart from all of its predecessors is that it marks the first time that NVIDIA has brought one of their modern, high-end compute-centric GPUs to the Titan family, and what that means for developers and users alike. NVIDIA’s massive GV100 GPU, already at the heart of the server-focused Tesla V100, introduced the company’s Volta architecture, and with it some rather significant changes and additions to NVIDIA’s compute capabilities, particularly the new tensor core. And now those features are making their way down into the workstation-class (and aptly named) Titan V.
NVIDIA GPU Specification Comparison | ||||||
Titan V | Titan Xp | GTX Titan X (Maxwell) | GTX Titan | |||
CUDA Cores | 5120 | 3840 | 3072 | 2688 | ||
Tensor Cores | 640 | N/A | N/A | N/A | ||
ROPs | 96 | 96 | 96 | 48 | ||
Core Clock | 1200MHz | 1485MHz | 1000MHz | 837MHz | ||
Boost Clock | 1455MHz | 1582MHz | 1075MHz | 876MHz | ||
Memory Clock | 1.7Gbps HBM2 | 11.4Gbps GDDR5X | 7Gbps GDDR5 | 6Gbps GDDR5 | ||
Memory Bus Width | 3072-bit | 384-bit | 384-bit | 384-bit | ||
Memory Bandwidth | 653GB/sec | 547GB/sec | 336GB/sec | 228GB/sec | ||
VRAM | 12GB | 12GB | 12GB | 6GB | ||
L2 Cache | 4.5MB | 3MB | 3MB | 1.5MB | ||
Single Precision | 13.8 TFLOPS | 12.1 TFLOPS | 6.6 TFLOPS | 4.7 TFLOPS | ||
Double Precision | 6.9 TFLOPS (1/2 rate) |
0.38 TFLOPS (1/32 rate) |
0.2 TFLOPS (1/32 rate) |
1.5 TFLOPS (1/3 rate) |
||
Half Precision | 27.6 TFLOPS (2x rate) |
0.19 TFLOPs (1/64 rate) |
N/A | N/A | ||
Tensor Performance (Deep Learning) |
110 TFLOPS | N/A | N/A | N/A | ||
GPU | GV100 (815mm2) |
GP102 (471mm2) |
GM200 (601mm2) |
GK110 (561mm2) |
||
Transistor Count | 21.1B | 12B | 8B | 7.1B | ||
TDP | 250W | 250W | 250W | 250W | ||
Manufacturing Process | TSMC 12nm FFN | TSMC 16nm FinFET | TSMC 28nm | TSMC 28nm | ||
Architecture | Volta | Pascal | Maxwell 2 | Kepler | ||
Launch Date | 12/07/2017 | 04/07/2017 | 08/02/2016 | 02/21/13 | ||
Price | $2999 | $1299 | $999 | $999 |
Our traditional specification sheet somewhat understates the differences between the Volta architecture GV100 and its predecessors. The Volta architecture itself sports a number of differences from Pascal, some of which we’re just now starting to understand. But the takeaway from all of this is that the Titan V is fast. Tap into its new tensor cores, and it gets a whole lot faster; we’ve measured the card doing nearly 100 TFLOPs. The GV100 GPU was designed to be a compute monster – and at an eye-popping 815mm2, it’s an outright monstrous slab of silicon – making it bigger and faster than any NVIDIA GPU before it.
That GV100 is appearing in a Titan card is extremely notable, and it’s critical to understanding NVIDIA’s positioning and ambitions with the Titan V. NVIDIA’s previous high-end GPU, the Pascal-based GP100, never made it to a Titan card. That role was instead filled by the much more straightforward and consumer-focused GP102 GPU, leading to the resulting Titan Xp. Titan Xp itself was no slouch in compute or graphics, however it left a sizable gap in performance and capabilities between it and the Tesla family of server cards. By putting GV100 into a Titan card, NVIDIA has eliminated this gap. However it also changes the market for the card and its expectations.
The Titan family has already been pushing towards compute for the past few years, and by putting the compute-centric GV100 into the card, NVIDIA has essentially ushered that transition to completion. The Titan V now gets all of the compute capabilities of NVIDIA’s best GPU, but in turn it’s more distant than ever from the graphics world. Which is not to say that it can’t do graphics – as we’ll see in detail in a bit – but this is first and foremost a compute card. In particular it is a means for NVIDIA to seed development for the Volta architecture and its new tensor cores, and to give its user base a cheaper workstation-class alternative for smaller-scale compute projects. The Titan family may have started as a card for prosumers, but the latest Titan V is more professional than any card before.
Putting this into context of what it means for existing Titan customers, and it means different things for compute and graphics customers. Compute customers will be delighted at the performance and the Volta architecture’s new features; though they may be less delighted at the much higher price tag.
Gamers on the other hand are in an interesting bind. Make no mistake, the Titan V is NVIDIA’s fastest gaming card to date, but as we’re going to see in our benchmarks, at least right now it’s not radically ahead of cards like the GeForce GTX 1080 and its Titan Xp equivalent. As a result, you can absolutely game on the card and boutique system builders are even selling gaming systems with the cards. But as we’re going to see in our performance results, the performance gains are erratic and there are a number of driver bugs that need squashed. The end result is that the messaging from NVIDIA and its partners is somewhat inconsistent; the $3000 price tag and GV100 GPU scream compute, but then there’s the fact that it does have video outputs, uses the GeForce driver stay, and is NVIDIA’s fastest GPU to date. I expect interesting things once we have proper consumer-focused Volta GPUs from NVIDIA, but that is a proposition or next year.
Getting down to the business end of things, let’s talk about today’s preview. In Greek mythology Titanomachy was the war of the Titans, and for our first look at the Titan V we’re staging our own version of Titanomachy. We’ve rounded up all four of the major Titans, from the OG GTX Titan to the new Titan V, and have tested them on a cross-section of compute, gaming, and professional visualization tasks in order to see what makes the Titan V tick and how the first graphics-enabled Volta card fares. Today’s preview is just that, a preview – we have even more benchmarks cooking in the background, including some cool deep learning stuff that didn’t make the cut for today’s article. But for now we have enough data pulled together to see how NVIDIA’s newest Titan compares to its siblings, and why the Volta architecture just may be every bit as big of a deal as NVIDIA has been making of it.
111 Comments
View All Comments
mode_13h - Wednesday, December 27, 2017 - link
I don't know if you've heard of OpenCL, but there's not reason why a GPU needs to be programmed in a proprietary language.It's true that OpenCL has some minor issues with performance portability, but the main problem is Nvidia's stubborn refusal to support anything past version 1.2.
Anyway, lots of businesses know about vendor lock-in and would rather avoid it, so it sounds like you have some growing up to do if you don't understand that.
CiccioB - Monday, January 1, 2018 - link
Grow up.I repeat. None is wasting millions in using not certified, supported libraries. Let's avoid talking about entire frameworks.
If you think that researches with budgets of millions are nerds working in a garage with avoiding lock-in strategies as their first thought in the morning, well, grow up kid.
Nvidia provides the resources to allow them to exploit their expensive HW at the most of its potential reducing time and other associated costs. Also when upgrading the HW with a better one. That's what counts when investing millions for a job.
For you kid's home made AI joke, you can use whatever alpha library with zero support and certification. Others have already grown up.
mode_13h - Friday, January 5, 2018 - link
No kid here. I've shipped deep-learning based products to paying customers for a major corporation.I've no doubt you're some sort of Nvidia shill. Employee? Maybe you bought a bunch of their stock? Certainly sounds like you've drunk their kool aid.
Your line of reasoning reminds me of how people used to say businesses would never adopt Linux. Now, it overwhelmingly dominates cloud, embedded, and underpins the Android OS running on most of the world's handsets. Not to mention it's what most "researchers with budgets of millions" use.
tuxRoller - Wednesday, December 20, 2017 - link
"The integer units have now graduated their own set of dedicates cores within the GPU design, meaning that they can be used alongside the FP32 cores much more freely."Yay! Nvidia caught up to gcn 1.0!
Seriously, this goes to show how good the gcn arch was. It was probably too ambitious for its time as those old gpus have aged really well it took a long time for games to catch up.
CiccioB - Thursday, December 21, 2017 - link
<blockquote>Nvidia caught up to gcn 1.0!</blockquote>Yeah! It is known to the entire universe that it is nvidia that trails AMD performances.
Luckly they managed to get this Volta out in time before the bankruptcy.
tuxRoller - Wednesday, December 27, 2017 - link
I'm speaking about architecture not performance.CiccioB - Monday, January 1, 2018 - link
New bigger costier architectures with lower performance = failtuxRoller - Monday, January 1, 2018 - link
Ah, troll.CiccioB - Wednesday, December 20, 2017 - link
Useless cardVega = #poorvolta
StrangerGuy - Thursday, December 21, 2017 - link
AMD can pay me half their marketing budget and I will still do better than them...by doing exactly nothing. Their marketing is worse than being in a state of non-existence.